Reading Time : 1 Mins
The Conundrum of Using Rule-Based vs. Machine Learning Systems
Would you like to know more about rule-based systems benefits or the limitations of machine learning (ML) systems? Or maybe you‘re looking for a guide on how to integrate these two concepts into your business. If so, then you‘ve come to the right place.
Machine learning? Self-learning systems? Sounds great. But scary at the same time. You keep asking: What are rule-based and machine learning or self-learning systems? And – is there a better choice for my project or application? You’re not alone. There is so much hype about rule vs. a machine that it can be hard to distinguish what’s true, relevant, helpful, and definitely hype.
But is the adoption based on actual needs or embracing state-of-the-art technology just for the sake of it? What happens to technologies such as rule-based systems, which were in vogue before Artificial Intelligence systems such as machine learning came into existence? Should all organizations shift to Machine Learning for better results, or can they continue to use rule-based systems?
In this article, let us discuss rule-based systems, machine learning, or self-learning systems and discuss the advantages, limitations, and the business needs to apply them.
- Introduction to rule-based systems
- What is a rule-based system?
- Advantages of rule-based systems
- Limitations of rule-based systems
- Introduction to machine learning and self-learning systems
- What is a machine-learning system? (or Self-learning system)
- Advantages of machine-learning system
- Limitations of machine-learning system
- Rule-based systems: Should you ignore them
- Is Machine Learning the way forward?
Ok, let’s get started!
Rule-Based Vs. Machine Learning Systems
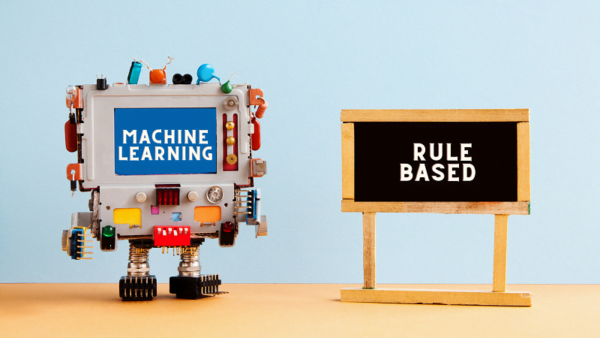
It’s a big decision for any company to make. When you choose how to automate your business problems, you can either use rule-based systems or machine learning systems. Each offers its own strengths and weaknesses.
The choice depends on the type of process you want to automate and your ultimate goal.
Let’s first understand these two systems better, shall we?
Introduction to Rule-based systems
Rule-based systems are computer programs that use if-then rules to make decisions and perform tasks. They have been around for decades, and they have revolutionized the way businesses operate in many ways. This is why it may be hard to believe that we still rely heavily on rule-based systems to make decisions, even with all of the advances in machine learning.
As much as rule-based systems seem like the obvious answer to a problem, they don’t always solve the problem optimally. This is because they are only as good as the quality of their rules, which are not always accurate or comprehensive. In fact, one of the main reasons rule-based systems are so popular is that when you have a very specific problem with a small set of options, rule-based systems can be very effective and efficient.
What is a Rule-based system?
Rule-based systems are a popular way of processing data. They use an if-this-then-that structure to take known information, process it, and develop a result. These systems are fast and easy to build, but because they rely on hardcoded rules and inference, they’re rarely accurate outside of their domain of expertise.
When you program the system to make decisions based on a certain set of rules, they are called rule-based systems. Human experts build rule-based systems with in-depth domain knowledge to guarantee the best possible outputs. Hence, they are expert-driven systems.
For example, when a bank receives an application for a loan from someone, the bank can use a simple rule like “If the applicant’s age is less <= than 50 and his income is >= $60000 annually,” the bank can approve the loan. Of course, this is a simple rule, and rule-based systems can be built into much more complex rules to qualify the applicant and decide whether to approve or reject the request.
As mentioned above, these rules are built by humans who are domain experts. The business knowledge they bring into making these rules is extremely critical for the system to accept inputs and provide outputs accordingly.
Advantages of rule-based systems
Rule-based systems are much easier to understand, modify and maintain than machine learning systems. In most cases, the rules are stored in a database, which can be modified by a business analyst or IT professional without any programming.
For example, if you want your chatbot to offer a coupon to customers who say, “I want to cancel my order,” you can just add that rule to the system’s database without having to write any code. Let’s look at a few of the advantages and disadvantages of rule-based systems.
1. Rule-based systems are easy to understand and interpret:
This is in stark contrast to a machine learning system that may be programmed by one person and implemented by another, who may not necessarily understand the details of how the machine learning system works.
2. Quick implementation:
A rule-based system can be implemented relatively quickly compared to a machine learning system, which can take months to build, test, and implement.
3. Easy modification:
A rule-based system can be modified quickly since the modifications only require changes to the rules themselves.
4. Durable in nature:
Rule-based systems are more durable; They do not change the “conditioning” of the system due to changes in the environment.
5. Compatible with ML/AI:
When combined with machine learning and AI methods, rule-based systems may increase their efficiency and effectiveness by combining different approaches.
Limitations of rule-based systems
The kinds of problems that rule-based systems solve haven’t disappeared, but they have been overshadowed by other types of problems. The main ones are as follows:
1. Problems with a vast number of variables:
When hundreds or thousands of variables are involved in any one decision, it can be hard for humans to formulate an exhaustive set of rules. This is where machine learning shines because it can easily incorporate all those variables without becoming unwieldy.
2. Problems with many constraints:
If your problem involves a lot of exceptions and exceptional cases that must be followed, you might have trouble writing rules that cover them all. Machine learning handles constraints much better and makes fewer mistakes than rule-based systems do.
3. Limited intelligence:
The ability to make decisions is limited by what we have explicitly programmed. Therefore, our ability to achieve intelligent behavior is limited by something that is not fully known at design time. If we do not know what rules need to be created in advance, they cannot be written into the system.
Introduction to Machine learning and Self-learning systems
Over the last decade, we have seen a transition to systems using machine learning algorithms to make decisions. Originally, the use of machine learning was restricted to applications such as image recognition and speech recognition, but it has now spread to all kinds of digital systems.
But what is a machine learning system or a self-learning system? Keep reading.
What is a Machine-learning system? (or Self-learning system)
Machine learning systems examine large amounts of past data and make decisions based on their learning from the data. For instance, in the loan application example above, a machine learning system can see that a loan applicant whose age is <=50 and income >=$60000 can be approved based on the vast number of applicant data from the past.
The important point to note here is that no one needs to tell the information above to the Machine Learning-based system. The software can make this logical deduction on its own by simply analyzing the data and looking for correlations.
The biggest difference between rule-based systems and self-learning systems is that humans manually program rule-based systems, whereas machines automatically train self-learning systems. In other words, self-learning systems learn from experience rather than being explicitly told what to do by humans.
Self-learning systems rely on machine learning algorithms to identify patterns from historical data. The system does not explicitly know which features are essential but can learn from data and predict future behavior based on historical information. In this case, if our goal were to identify frequent travelers, we would train the model using historical data (e.g., demographic information) that includes attributes like age, gender, education level, and purchase history. Based on these attributes, we could create labels such as “frequent traveler” or “occasional traveler” and train the model accordingly.
In short, a machine learning system learns by itself from patterns within the data fed to it, while rule-based systems use rigid “If-else” rules that have to be hand-crafted.
Advantages of Machine-learning system
Machine learning systems are great for problems where it’s hard to write rules or come up with algorithms. Let’s take a look at a few of the advantages and disadvantages of machine learning systems.
1. Self-learning systems:
Machine learning systems can learn from past data and adapt to new situations by themselves, whereas rule-based systems require human intervention for any changes.
2. Handling more complex problems:
Machine learning is excellent at finding patterns in data, but it can also find patterns that don’t exist. This is a big problem for businesses that rely on machine learning to identify fraudulent transactions or suspicious activities. Such detection rates are not accurate enough for the models to be used in production.
3. Performing better with less human interaction than rule-based systems:
The significant advantage is that the machine learning system doesn’t need to be explicitly programmed for performing a task like a rule-based system.
4. Adapting over time (via continuous learning) to changes in data and environment:
A machine-learning system will adapt and evolve as new data becomes available. It doesn’t require human intervention to update or refine the rules it uses. And because it’s constantly learning from new data, its accuracy improves over time.
Limitations of machine-learning system
Before spending too much time building a machine-learning system, there are some limitations you should be aware of:
1) Machine-learning systems need to see a large number of inputs before they can accurately reproduce outputs on their own. If your data set is small or doesn’t include many different examples, it won’t help much in training your system. In this case, there’s not enough information for it to learn from.
2) Machine-learning systems can only learn from data they’ve seen before. A data modeling trained on photos of cats probably won’t recognize photos of dogs very well — even if they’re very similar. Machine learning models only know what you teach them; there is no room for human intuition. If you train one on old images of dogs and cats, then show it one with both in the same photo, then there’s no guarantee that it will correctly identify both subjects in the image.
3) Machine-learning systems are not intelligent in the sense that humans are. They have no common sense, and they do not “understand” what they are doing in any meaningful way. They cannot adapt to new situations or solve problems for which they have not been trained.
Rule-based systems: Should you ignore them
Based on what we have seen so far, it looks like machine learning is the way to go because it doesn’t need any human interaction and learns itself from “past data” to make decisions.
But from where can Machine learning systems get this “past data,” which is critical to the model’s behavior? An existing rule-based system can provide the same. The data from the rule-based system can come in handy in increasing the accuracy of the machine learning algorithm. A 50% accuracy (which is the same as a coin toss) is something you can expect from a machine learning model that uses the rule engine’s data.
However, rule-based systems are prone to human error, and the integration of rules can be time-consuming and expensive. Complex and too many rules also contribute to performance degradation. As rules become stricter, there is a high possibility of losing out on good customers.
Having said that, rule-based systems can execute decisions much faster with proper training. They are reliable. Rule-based systems can be valuable when exact answers are required, and the number of rules and options is relatively simple. The output of a rule-based system is easy for a human to debug.
Is Machine Learning the way forward?
The expectation that comes with using machine learning is that they are artificial intelligence systems that provide high levels of accuracy compared to humans. This notion leads to “machines replacing humans,” “reducing human effort,” “time savings,” and so on. But building a machine learning system is no joke, and a wrongly constructed machine learning system can cost an organization dearly on all fronts, including cost, effort, and usage.
While machine learning systems provide significant advantages over the capabilities of a rule-based system, it would be a mistake to consider machine learning as the silver bullet to all of your problems.
Machine learning models take time to understand and learn from data. A machine learning model is only as good as the data it absorbs, and it can take months for the system to be ready to replace rules. There is no point in expecting “super accuracy” from a machine learning model overnight or complaining that it is underperforming compared to your current rule-based engine.
Conclusion
A good approach when transitioning from rule-based systems to machine learning is to run rules in parallel with machine learning. This helps to compare results over time and decide when to replace machine learning in the place of your rule-based system.
You can also consider operating rules and machine learning systems in tandem, which would be more beneficial to the organization than replacing rules entirely. Machine learning is not meant to substitute humans but augment what humans are capable of. Results from the rightly built machine learning model can imitate human capabilities, completely complementing human efforts and helping in increasing their productivity.
While machine learning’s strength is in the amount of data it can analyze and track in real-time, a human’s strength is in providing context and intuition in analyzing outliers and other edge-case scenarios.
It is important to consider the domain expertise that a human brings to the building rules that makes rule-based systems successful.
We hope you like this article and learn how machine learning is an intrinsic part of data science! Book a discovery service with our data architects today and get ahead of the competition. Make it simple & make it fast.
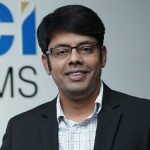
Bibliophile, Movie buff & a Passionate Storyteller. President @ Zuci systems
Share This Blog, Choose Your Platform!
Related Posts