Reading Time : 1 Mins
Artificial Intelligence (AI) and Machine Learning (ML) Use Cases in Banking
I write about fintech, data, and everything around it
This is a blog about the future of AI and Machine Learning, and how leading technologies will shape the future of the banking and finance sector
Did you know that JPMorgan, Bank of America, and Morgan Stanley have heavily invested in machine learning to develop automated investment advisors?
It doesn’t stop there. Artificial Intelligence (AI) and Machine Learning (ML) are being extensively used in the banking sector for financial monitoring, risk management, marketing, retention, data management, process automation, algorithmic trading, and whatnot.
A recent survey of 34 major banks across several geographies (US, EU, Singapore, Africa, Australia, India) found that 27 out of these 34 banks have implemented artificial intelligence in front-office functions, including as chatbots and virtual assistants. Some of the most prominent banks in this space across regions are Bank of America, OCBC, ABN Amro, YES BANK, etc.
In the US, all major banks are experimenting with artificial intelligence to improve their business in at least four ways: to make customer interactions smoother; reduce fraud and money laundering; improve tax reporting; and to automate regulatory reporting for compliance with the law.
Let us look at Some of the ways in which AI and ML will Shape the Banking and Finance sector:
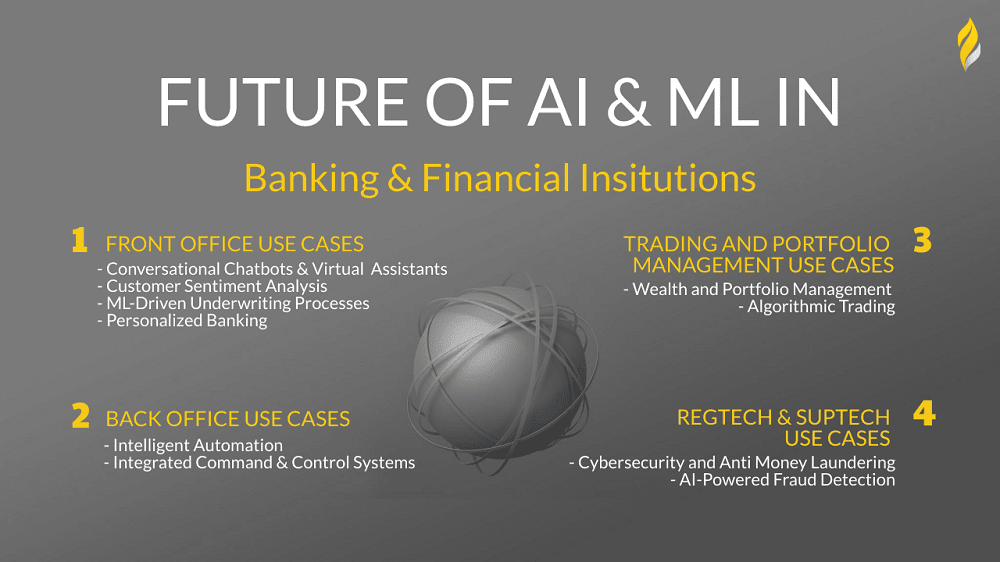
AI & ML in Banking: Front Office Use Cases
Artificial intelligence and machine learning are going to be a critical part of the future of finance. They will help banks to analyze data, predict customer behavior, and customize financial services.
We are going to see a fundamental shift in how financial services operate towards data-driven banking. In the next five years, many banking processes will change thanks to artificial intelligence and machine learning technologies.
-
Conversational Chatbots & Virtual Assistants
Consumers want to interact with their banks in a more conversational way. They want the same level of customer service that they receive from the likes of Amazon, Netflix and Uber. The advent of chatbots and virtual assistants are helping to make this possible in banking.
Just as consumers have come to expect a higher level of customer service from other industries, they also expect it from their banks. AI-powered chatbots and virtual assistants can provide around-the-clock advice to customers on matters such as bank account balances and other transactions. They can also allow customers to send money using conversational language.
-
Customer Sentiment Analysis
With the advent of big data and machine learning, customer sentiment analysis is a key area for banks to apply AI. Banks already have access to a wealth of data about their customers, but much of this is unstructured and therefore difficult for computers to understand. AI, however, can make sense of this data and draw new insights from it.
For example, when a customer calls the help desk with a problem, an AI system can analyse what they say and identify the emotions they are expressing in real time. This allows banks to determine whether their staff are resolving issues effectively, as well as taking action if they don’t soothe their customers’ concerns.
These systems can also be used to analyse social media posts and other customer feedback. By identifying patterns in what people say about their bank online, banks can use AI to predict how customers will react to particular events — such as the launch of a new product or service — or even to changes in market conditions that could affect them.
-
ML-Driven Underwriting Processes
While analyzing the creditworthiness of a customer, if they do not have a credit history with the bank, it can be annoying. Big data and ML analyze 10,000+ data points which help assess how credit-worthy they are. Thereby, it makes it possible to give pre-approved loans to a huge mix of customers, even students and those who are self-employed.
Even in the case of corporate lending, AI based underwriting will be able to simplify this complex process, analyze market trends, identify risks in lending, behavior in the future, the likelihood of fraud, etc.
Here is a short explainer video of our solution HALO – An analytics product with AI/ML capability, aimed at data-driven automated credit decisions for banks and financial institutions. With HALO, lending businesses can now reduce the overall credit cost by improving the quality of loan disbursals. Enter the world of AI-Powered lending – HALO.
-
Personalized Banking
“The biggest benefit of AI and Machine Learning is Personalization. I believe that a bank of the future should be able to provide insights, alerts and recommendations tailored to an individual’s own financial goals, based on their spending habits, current savings and investment plans, health insurance portfolios and other factors.
At present, AI is in early stages of development and implementation in the banking sector. For example, some banks are already using predictive analytics to determine and predict customers’ financial needs.
This is just the first step towards personalized banking, as banks need to know how customers want to be addressed before they can offer them personalized services. A lot of work needs to be done here. Banks require a ton of data that’s fragmented across various systems and departments. This needs to be brought together with the help of Artificial Intelligence so it can mine this information and deliver relevant insights or advice for a customer.
Here is a quick insight on “Personalized Banking” from our Senior Manager – Business Intelligence & Analytics, Rajkumar Purushothaman for building the bridge between digital and physical banking.
AI & ML in Banking: Back Office Use Cases
-
Intelligent Automation
ML solutions will replace a lot of manual work by automating manual tasks with the help of intelligent process automation. Some of the examples of process automation in the banking sector that are possible are automating paperwork, chatbots, employee training gamification, etc. It not only reduces costs, but it also enhances the experience of the customer, while also allowing banks to scale their operations.
Check out some of the use cases which we help our banking partners with.
-
Integrated Command & Control Systems
In today’s financial services world, banks and other financial institutions have to deal with not only their own data but also the data of third parties. The problem with this is that it creates silos of data that are not integrated into a single source of truth system. This is a big issue because it means that banks are not able to analyze all the data they have in order to make better decisions.
In order to create these single source of truth systems, data engineering and machine learning algorithms can be used to integrate the different sources of data together. This is called as Integrated Command & Control Center. The benefit of this approach is that it allows banks to make better decisions based on all their available data rather than just a subset.
AI & ML in Banking: Trading and Portfolio Management Use Cases
-
Wealth and Portfolio Management
The AI-based systems can even determine who its potential investors could be based on their salary and spending behavior. It is also capable of assessing market trends and choosing good funds based on their portfolio. The best part about this is that it can be done virtually, without having to step foot in your branch.
Mutual funds? Fixed deposits? All of this and more can be done from the comfort of your home. You can thank AI and ML for this.
-
Algorithmic Trading
Clearly, machine learning is the future of trading. Algorithmic trading — trading platforms that use computers to make trades — can be more efficient and accurate than real live traders and have many other advantages.
While most people are familiar with algorithmic trading, we’re also seeing the rise of machine-learning algorithms in banking. Machine-learning algorithms can work on a massive scale — they don’t need a data scientist to tell them what they’re supposed to do. They can look at millions of data points at once and draw conclusions that are much more accurate than regular traders ever could be.
By using machine learning, banks can widen their horizons beyond traditional risk models and focus on the areas that are most profitable right now.
AI & ML in Banking: Regulatory compliance (RegTech) & Supervision (SupTech) Use Cases
-
Cybersecurity and Anti Money Laundering
An innovative integration of AI and machine learning into banking is sure to make banking more secure in the near future. The use of AI and ML will help the financial services industry to enhance their cybersecurity and anti-money laundering operations.
A large number of banks are implementing AI and ML technology to identify any suspicious or unusual transaction that may be related to money laundering activities. It is expected that the use of AI and ML technologies will significantly increase in the coming years.
The integration of these technologies is sure to provide a more streamlined service, saving time, money, and manpower. Moreover, it will also help in strengthening the security systems of banks, businesses and individuals to prevent cyber attacks on their institutions, networks, and accounts.
-
AI-Powered Fraud Detection
ML algorithms have the capacity to detect fraud by going through millions of data points. It improves the quality of real-time approvals while reducing the number of false rejections. If there is any suspicious behavior from certain bank accounts, it will identify them in real-time instead of detecting them after the crime.
For every $1 that financial institutions lose to frauds, the recovery costs are at least $2.92. This is where AI and ML can create a huge dent in the banking sector. Although banks have monitoring systems, they are usually based on previous payment data. The ML-powered algorithm uses vast amounts of credit card transaction datasets and it can easily label events as fraud versus non-fraud.
Conclusion:
Although AI and ML are still in their infancy stages in the banking sector, we can certainly hope that it will see even wider adoption. Thankfully, financial institutions are also beginning to understand the kind of impact that technologies such as AI and ML bring in. But the truth is that most banks follow rigid systems that pose significant operational and organizational challenges in becoming a technologically-powered organization. To overcome this, banks have to trust in these technologies and prepare themselves to adopt them.
If you are looking to power your financial organization with AI and ML, call the experts at Zuci. Schedule a 30-mins call now.
Related Posts
Related Posts