Reading Time : 3 Mins
Data Science in Healthcare Industry: Benefits, Strategies, Applications, Tools, and Future Trends
I write about fintech, data, and everything around it
Curious about how data science can help the healthcare industry? This blog explains all about data science technology with 13 use cases of practical data science applications for the healthcare industry.
Data science is a multidisciplinary field that uses scientific methods, data mining techniques, machine-learning algorithms, and big data to extract knowledge and insights from a wide range of structured and unstructured data.
The healthcare industry produces massive amounts of valuable data on patient demographics, treatment plans, medical examination results, insurance, etc. Data science and big data analytics can provide practical insights and assist in the decision-making process for strategic healthcare decisions. It contributes to developing a comprehensive picture of patients, customers, and clinicians. Data-driven decision-making opens up new avenues for improving healthcare.
The article examines data science’s current state and prospects in healthcare, highlighting the benefits, describing the frameworks and techniques used, summarising the current challenges, and discussing viable solutions.
Let’s get started.
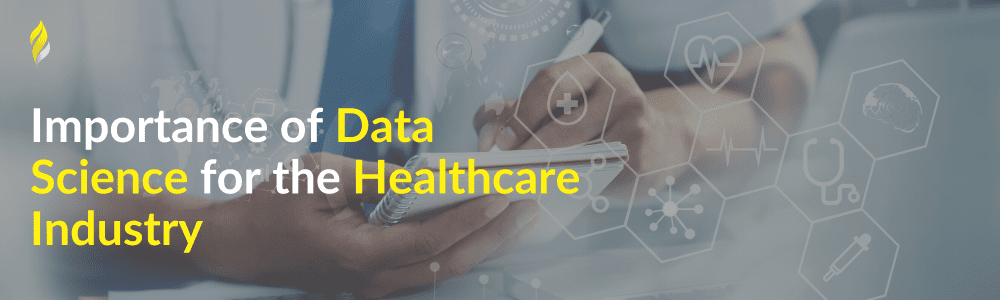
Importance of Data Science in the Healthcare Industry
According to one study, each human body generates 2 terabytes of data per day. This information includes brain activity, stress, sugar, heart rate, and many other things. To manage and maintain such massive amounts of data, we now have more advanced technologies, one of which is data science. It aids in tracking patients’ health by utilizing recorded data.
Thanks to the data science applications in healthcare, it is now possible to detect disease symptoms at an early stage. Doctors can also monitor patients’ conditions from remote locations thanks to the development of various ground breaking tools and technologies.
Previously, doctors and hospital administration could not handle a large number of patients at the same time. And because of a lack of proper treatment, the patient’s conditions deteriorated.
With data science applications in healthcare, the situation has changed. Data science and Machine Learning applications can notify doctors of patients’ health conditions via wearable devices. The hospital administration can dispatch junior doctors, assistants, or nursing staff to these patients’ homes.
Hospitals can also install various diagnostic equipment and devices for these patients. These data Science-based devices can collect data from patients such as heart rate, blood pressure, temperature, and so on. Upgrades and notifications in mobile applications provide doctors with real-time patient health data. They can then diagnose the conditions and help junior doctors or nurses administer specific treatments to patients at their homes. This is how data science can aid in patient care by utilizing technology.
Benefits of Data Science in the Healthcare Industry
For healthcare, data science is now an essential component and determinant that has changed the industry. Many facilities and processes have advanced as a result of data science, technological know-how tools, and techniques. It has accelerated treatment and diagnosis. As an outcome, the workflow of the healthcare device improves. The following are the benefits of data science in healthcare:
- It aids in the proper handling of emergency situations.
- It shortens the treatment time for patients.
- It provides prompt and appropriate treatment.
- It improves the efficiency of the healthcare workflow.
- It aids in lowering the risk of failure when treating any affected individual.
Application of Data Science in Healthcare Industries
Data science has numerous applications in the healthcare industry, revolutionizing the way healthcare providers operate, researchers conduct studies, and patients receive care. Here are some key applications of data science in healthcare:
Predictive Analytics: Data science techniques can be used to analyze large volumes of healthcare data, including patient records, demographics, and medical histories, to identify patterns and predict outcomes. This helps in predicting disease progression, identifying high-risk patients, and suggesting personalized treatment plans.
Disease Diagnosis and Prognosis: Data science algorithms can aid in the early detection and accurate diagnosis of diseases. By analyzing patient symptoms, medical images, and lab results, data-driven models can provide insights that assist healthcare professionals in making informed decisions about diagnosis and prognosis.
Personalized Medicine: Data science enables the development of personalized treatment plans based on individual patient characteristics, such as genetic makeup, medical history, lifestyle factors, and environmental influences. This approach can optimize treatment efficacy and minimize adverse effects.
Drug Discovery and Development: Data science techniques are employed in drug discovery and development processes. Machine learning algorithms can analyze vast amounts of data, including molecular structures, genetic data, and clinical trial results, to identify potential drug candidates and predict their efficacy.
Health Monitoring and Wearable Devices: Data science plays a crucial role in monitoring health and wellness through wearable devices such as fitness trackers, smartwatches, and remote patient monitoring systems. These devices collect and analyze data on heart rate, sleep patterns, physical activity, and other health indicators to provide real-time insights and early detection of abnormalities.
Health Records and Clinical Decision Support: Data science techniques can be used to manage and analyze electronic health records (EHRs) to extract valuable insights, identify trends, and support clinical decision-making. This includes developing clinical decision support systems that provide evidence-based treatment recommendations.
Public Health Surveillance: Data science helps in monitoring public health by analyzing large-scale datasets, such as disease surveillance data, environmental data, and social media data. It enables early detection of disease outbreaks, tracking the spread of infectious diseases, and implementing effective public health interventions.
Healthcare Operations and Resource Optimization: Data science techniques can improve healthcare operations by analyzing data on patient flow, resource utilization, and wait times. This information helps optimize scheduling, staffing, and resource allocation, ultimately improving patient care and reducing costs.

Why Should Organisations Start Thinking About a Data Department?
The future of every business is data-driven. Data is one of the most valuable resources for any organization and is the oil you need to run the whole company. But why do you need a data department? What will a data department do that an IT department isn’t already doing? This blog explains all your questions.
5 Strategies to Advance Big Data and Data Science in the Healthcare Sector
The healthcare sector is one of the most data-rich industries, with more than $2 trillion in data generated yearly. The healthcare industry is also one of the most advanced in big data and analytics.
Big data and analytics are now essential to the success of any business, especially healthcare companies. Here are five strategies that you can use to advance big data and data science in the healthcare sector:
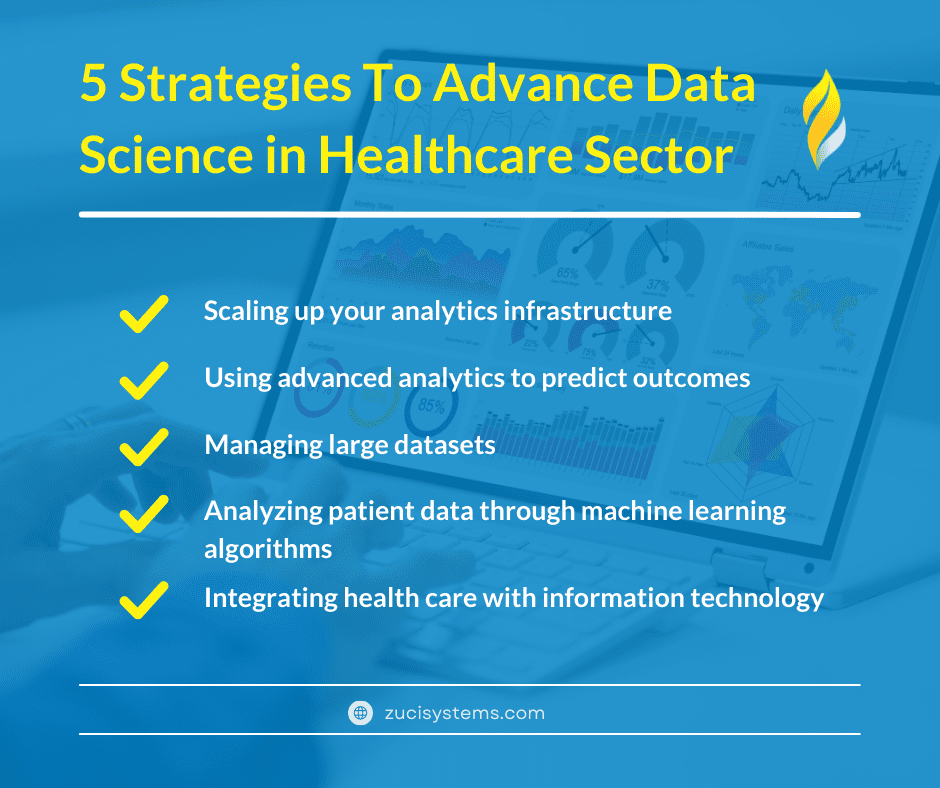
1. Scaling up your analytics infrastructure
The key challenge for any organization that wants to use big data and analytics is how they will scale their solutions. As the amount of data being analyzed increases, so does the need for more powerful computing resources. This means that you will need more servers and storage space than you initially anticipated so that you can keep up with all of the new data coming into your system every day.
2. Using advanced analytics to predict outcomes
One of the most important things you can do to advance big data and data science in the healthcare sector is to use advanced analytics to predict outcomes. This is a key component of determining the best treatment or care plan for your patients. By combining individual records with historical data, you can determine how individuals respond to treatments and ultimately improve patient outcomes.
3. Managing large datasets
Another important strategy to advance big data and data science in the healthcare sector is managing large datasets. Large datasets mean that you’re able to analyze more details about each patient’s condition, which allows you to make better diagnoses and provide better treatments. It also lets you see patterns in disease progression that were previously invisible.
4. Analyzing patient data through machine learning algorithms
One of the most promising uses of big data is to improve the quality of care provided to patients. Machine learning algorithms allow medical professionals to develop accurate diagnoses based on certain patterns in patient medical records. These algorithms also help identify disease trends and predict future health outcomes, enabling doctors to make better treatment decisions. For example, researchers from Stanford University developed an algorithm that could predict which patients would respond well to an experimental drug by analyzing their genetic profiles. The researchers then tested their predictions on more than 600 patients with various types of cancer. The test results proved extremely accurate — only one out of every 1,000 patients who would not respond well to the drug ended up being given it anyway.
5. Integrating health care with information technology
Integrating health care with information technology will help you analyze patient data in order to improve the quality of care delivered by doctors, nurses, and other healthcare professionals working in hospitals or clinics. This can be achieved by introducing artificial intelligence (AI) algorithms into hospital systems that analyze patient records and clinical notes for signs of disease or illness.
This approach would help reduce errors made by doctors because they could get accurate information about their patients’ conditions from their records instead of having to rely on guesswork or personal experience when making decisions about their treatment plans. It would also allow hospitals or clinics to tailor treatments based on an individual’s health history so they can address any existing medical conditions before they become serious problems that require emergency care.

Integrated Command & Control Center: Why does Every Organization Need This?
Have you ever imagined having a command & control center, right at your fingertips, where you could see an overview and complete 360-degree summary of your business operations in real-time? Well, today, it is no longer a need. It is a necessity. And this blog is to help you understand why every organization needs an Integrated Command & Control Center and the benefits of having one.
Future of Data Science in the Healthcare Industry
The future of data science in the healthcare industry is promising, with continued advancements and increasing adoption of data-driven approaches. Here are some key trends and possibilities for the future:
Precision Medicine: Data science will play a pivotal role in advancing precision medicine, which tailors treatment plans to individual patients based on their genetic makeup, lifestyle, and environmental factors. By integrating large-scale genomic data, clinical records, and other relevant data sources, data science techniques will enable more precise diagnosis, treatment selection, and monitoring of patients.
Real-time Monitoring and Predictive Analytics: With the growing availability of wearable devices and remote patient monitoring systems, data science will enable real-time monitoring and analysis of health data. Advanced predictive analytics algorithms will continuously assess data streams, detect anomalies, and alert healthcare providers to potential health risks, allowing for early intervention and improved patient outcomes.
Artificial Intelligence and Machine Learning: The integration of artificial intelligence (AI) and machine learning (ML) techniques will further enhance healthcare decision-making. AI-powered systems can analyze vast amounts of patient data, medical literature, and clinical guidelines to provide personalized treatment recommendations, assist in medical imaging analysis, and automate administrative tasks, thereby improving efficiency and accuracy.
Big Data and Integration: The healthcare industry generates enormous volumes of data from diverse sources such as electronic health records, genomics, wearables, social media, and public health databases. Data science will continue to evolve to handle these big data challenges, allowing for the integration and analysis of disparate datasets to uncover new insights, correlations, and trends.
Ethical Data Use and Privacy: As data science becomes more prominent in healthcare, the industry will face ethical considerations and privacy concerns. Striking the right balance between data utilization and patient privacy will be crucial. Data scientists, healthcare providers, and policymakers will need to work together to establish ethical frameworks, robust data governance practices, and stringent security measures to ensure the responsible and secure use of healthcare data.
Collaboration and Interoperability: The future of data science in healthcare will require enhanced collaboration and interoperability between different stakeholders. This includes interoperable data systems, standardized data formats, and collaborative platforms that enable data sharing and collaboration among researchers, clinicians, and data scientists to accelerate discoveries and improve patient care.
Augmented and Virtual Reality: Augmented reality (AR) and virtual reality (VR) technologies have the potential to transform healthcare education, surgical procedures, and patient engagement. Data science will be instrumental in analyzing and visualizing complex medical data, creating immersive AR/VR experiences, and optimizing training simulations for healthcare professionals.
13 Data Science Use Cases & Applications for the Healthcare Industry
Data science is used in a variety of fields in healthcare, including medical imaging, drug development, genetics, predictive diagnosis, and many others. We’ll go over each data science use case in healthcare with examples.

Healthcare Data Science Use Case #1: Medical Imaging
Medical imaging is the primary and most important application of data science in healthcare. Imaging techniques such as X-Ray, MRI, and CT scans are available. All of these techniques depict the inner workings of the human body.
Conventionally, doctors would manually examine these images for irregularities. However, it was frequently difficult to detect microscopic deformities, so doctors could not provide a proper diagnosis.
Deep learning technologies in data science have made it possible to detect such microscopic deformities in scanned images. It is possible to search for flaws in scanned images using image segmentation.
Healthcare Data Science Use Case #2: Genomics Data Science
Genomics is the study of genome sequencing and analysis. A genome comprises DNA and all of an organism’s genes. From completing the Human Genome Project, research has advanced rapidly and infiltrated the fields of data science and big data.
Before the accessibility of powerful computation, organizations spent significant time and money analyzing gene sequences.
However, with enhanced data science tools and advanced healthcare data science, it is now possible to examine and derive insights from human genes in much less time and at a much lower cost.
The primary objective of research scientists is to examine genomic strands for irregularities and defects. Then they look for links between genetics and a person’s health.
In general, data science is used by researchers to analyze genetic sequences and try to find a link between the variables contained within them and the disease.
Furthermore, genomics research includes finding the right drug, which provides a deeper understanding of how a drug reacts to a specific genetic issue. Bioinformatics is a relatively new field that incorporates data science and genetics.
Healthcare Data Science Use Case #3: Drug Discovery
Drug discovery is a highly technical field. Pharmaceutical companies rely heavily on data science to solve problems and develop improved patient drugs. Drug discovery turns out to be a time-consuming procedure that also involves significant financial investment and testing.
Machine Learning and data science algorithms are revolutionizing this process, providing extensive insights into optimizing and increasing prediction success rates.
Pharmaceutical companies use patient data insights such as mutation profiles and patient metadata. This data assists researchers in developing models and determining statistical relationships between the features.
Companies can then design drugs targeting vital mutations in the genetic sequences. Deep learning algorithms can also predict disease incidence in the human body.
Data science systems can also help simulate how drugs will act in the human body, eliminating the need for lengthy laboratory experiments.
Healthcare Data Science Use Case #4: Healthcare Predictive Analytics
Predictive Analytics in healthcare is one of the most widely discussed topics in health analytics. A predictive model utilizes historical data to learn from it, discover patterns, and make accurate predictions.
It discovers correlations and associations between symptoms, habits, and diseases and makes meaningful predictions.
Healthcare predictive analytics is helping to improve patient care, chronic disease management, and the efficiency of supply chains and pharmaceutical logistics.
Population health management is becoming a hot topic in predictive analytics. It is a data-driven method of disease prevention that focuses on diseases that are prevalent in society.
With the help of data science, hospitals can predict patient health deterioration and provide preventive measures and early treatment, reducing the risk of further deterioration of patient health.
Predictive analytics in healthcare is also helpful in tracking the logistic supply of hospitals and pharmaceutical departments.
Healthcare Data Science Use Case #5: Patient Health Monitoring
Data science is critical in IoT. (Internet of Things). These IoT devices, which are present as wearable devices, track the users’ heartbeat, temperature, and other medical parameters. Data science in healthcare is used to analyze the data that is collected.
Doctors can use analytical tools to monitor a patient’s circadian cycle, blood pressure, and calorie intake. A doctor can monitor a patient’s health using home devices and wearable monitoring sensors. Several systems for chronically ill patients track their movements, monitor their physical parameters, and analyze the patterns in the data.
It uses real-time analytics to predict whether the patient will have a problem based on their current condition. Furthermore, it assists doctors in making the necessary decisions to help patients in distress.
Healthcare Data Science Use Case #6: Disease Monitoring and Prevention
Data science is critical in monitoring patients’ health and notifying requisite steps to be taken to prevent possible diseases from occurring. Data Scientists use powerful healthcare predictive analytical tools to identify chronic diseases early on.
In many extreme situations, diseases are not detected at an early stage due to their ineligibility. This has a negative impact on not only the patient’s health but also the economic costs. As a result, data science healthcare plays a significant role in optimizing financial spending on healthcare.
In several cases, AI has played a significant role in identifying diseases at an initial stage. Researchers at Brazil’s University of Campinas have created an AI platform to detect the Zika virus using metabolic markers. Machine learning is being used by several other companies, including IQuity, to detect autoimmune diseases.
Healthcare Data Science Use Case #7: Offering Virtual Assistance
Data scientists have created an extensive virtual platform that assists patients with the help of disease predictive data modeling.
Patients can use these platforms to enter their symptoms and receive information and insight about the various potential diseases based on their confidence rate. Data science applications in healthcare help patients suffering from psychological issues such as depression, anxiety, and neurodegenerative diseases such as Alzheimer’s use virtual applications to assist them in their daily tasks.
Ada, a Berlin-based startup that predicts diseases based on the user’s symptoms, is a popular example of a virtual assistant. And Woebot, a Stanford University-developed chatbot that provides therapy treatments to patients suffering from depression.
Healthcare Data Science Use Case #8: Preventing Mistakes and Streamlining the Process of Medical Records Management
With the recent increase in health-related issues, more medical establishments have recognized the importance of building a system for proper medical record management.
Several organizations provide medical record management training. The only thing that matters is that the training you receive comes from an authentic and well-known organization and will assist you and your staff in performing your roles effectively. Training programs typically last six weeks. You must be extremely careful to ensure that all the information and protocols you’ve learned have been implemented correctly in your hospital or clinic.
When you have an electronic health record, you will better understand your patient’s needs. Patients’ current health status, drug prescriptions, and up-to-date information can be centralized. When medical records are in electronic form, it is also easier for other doctors and nurses to review them. When medical records are in electronic form, it is also easier for other doctors and nurses to check them.
Applying data science in healthcare will aid you in your record-keeping duties by organizing your medical records. Any of them can be downloaded from the Internet and installed on your computer or laptop. This is one of the most basic methods for avoiding medical record mismanagement. Such programs have various characteristics, so compare them carefully to choose the most appropriate software for your health institute.
Healthcare Data Science Use Case #9: Automatic Disease Detection via Wearable
The amount of data generated by the human body each day is two terabytes. We can now collect most of it thanks to technological advances, including information about heart rate, sleep patterns, blood glucose levels, stress levels, and even brain activity. With such a wealth of health data at their disposal, scientists are pushing the limits of health monitoring.
More common conditions, such as heart or respiratory diseases, can be detected and tracked using machine learning algorithms. Technology can detect the smallest changes in a patient’s health indicators and predict potential disorders by gathering and monitoring heart rate and breathing patterns. While 600,000 people in the United States die from sudden cardiac arrest each year, having the ability to predict the issue and send out timely alerts could save countless lives.
Healthcare Data Science Use Case #10: Developing More Effective Drugs
As the world’s population grows, new problems in the human body emerge regularly. Such a situation may occur due to a lack of proper food, chronic anxiety, pollution, physical illnesses, or other factors. Finding medicines or vaccines for diseases in a timely manner has now become a challenging task for medical research institutes. Because researchers must understand the characteristics of the causative agent to find a formula for a medicine, millions of test cases may be required. The researchers must then conduct additional tests on the formula after discovering it.
It used to take 10-12 years to go through the data of millions of test cases. However, with the help of various data science applications in healthcare, it has become a much easier process. Data through millions of test cases can be produced in months, if not weeks. It aids in determining the efficacy of a drug through data analysis. As a result, a successful vaccine or medicine can be released in less than a year. This is made possible by data science use cases in healthcare and Machine Learning. Both have revolutionized the pharmaceutical industry’s research and development sectors. Following that, we’ll look at how data science is used in genomics.
Healthcare Data Science Use Case #11: Improving Disease Diagnosis with Machine Learning
Machine learning owns the potential to transform clinical decision-making and prognosis. A doctor’s goal in medical diagnosis is to explain a patient’s symptoms by assessing the diseases causing them. On the other hand, existing machine learning approaches to diagnosis are solely associative, identifying conditions that are highly correlated with a patient’s symptoms. This inability to distinguish between correlation and causation can lead to suboptimal or dangerous diagnoses. Reframe diagnosis as a counterfactual inference task and develop counterfactual diagnostic algorithms to get around this.
Healthcare Data Science Use Case #12: Digital Pharmacies Automate Patient Adherence Programs
First and foremost, let us try to define pharmacy automation. It automates routine but time-consuming tasks in a pharmacy, such as medication dispensing, packaging, tracking, updating, and retrieval. It also includes managing customer information, medical history, and drug interactions. This advancement brought upon by the inventions and innovations in data science automates tasks that pharmacists have been conducting manually for a long time.
These automated solutions opted by most pharmacists have helped enhance the patient experience, avoid expensive and potentially dangerous errors, and enable independent pharmacists to operate more efficiently. You may free up time to focus on your patients’ health by automating your business’s health. It will be a win-win situation for everyone involved, from patients to pharmacy technicians to the pharmacists in charge.
Healthcare Data Science Use Case #13: Remote Patient Monitoring
The applications created with virtual assistance are an excellent example of data science in healthcare. Data Scientists have created comprehensive platforms that provide patients with personalized experiences. Data science-based medical applications (Healthcare apps) help patients identify diseases by analyzing symptoms. The patient only needs to enter their symptoms, and the application will predict the patient’s disease and condition. It will recommend precautions, medication, and treatment based on the patient’s condition.
Remote patient monitoring, i.e., remote patient management or RPM, is a method of healthcare delivery that uses the most recent advances in healthcare data science to gather patient data outside of traditional healthcare settings. However, don’t be led astray by the focus on technology: the most effective remote patient monitoring systems rely on today’s sleek, consumer-friendly data science healthcare personal tech products rather than the cold, isolating, wire-laden medical equipment of the past.
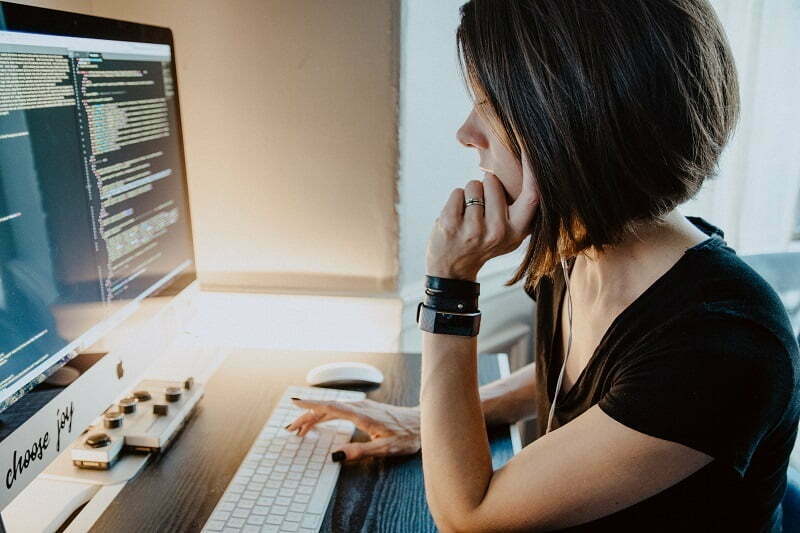
What is the Role of Machine Learning in Data Science?
Are you investing in ML and hiring more data scientists and machine learning engineers? Is there a lack of clarity on the role of machine learning and its place in the life cycle of a data science project? Here’s an attempt to resolve this uncertainty.
Data Science Tools for the Healthcare Industry
Data science tools in healthcare help health data scientists to use them and interpret insights that can help with new inventions. A few examples of some of the best data science tools for the healthcare industry are as follows –
- Hadoop by Apache
Hadoop is primarily used for the parallel processing of data distributed across multiple channels. Because it is a distributed file system, this data science in healthcare tool has the potential to analyze data from multiple nodes. Most healthcare organizations use this healthcare data science tool because it is open-source software.
- Statistical Analysis System
Statistical Analysis System is one of the immensely popular data science applications in healthcare due to its visually attractive representation of data analysis. Minute assessments of large healthcare datasets and filtering the most beneficial results enable healthcare data scientists to work on the problems in front of them.
Future Data Science Trends in the Healthcare Industry
Now that we have taken a detailed tour of the difference that data science has brought in the healthcare industry around the world, let’s now take a glimpse at the four factors that are driving dramatic improvement in the healthcare industry:
- Technological Innovations
- Need for digitalization
- High treatment costs
- The requirement for dealing with a large population
The healthcare industry is also facing challenges in terms of technology adoption and cost-effectiveness. Healthcare organizations have used various technologies such as electronic health records (EHR) and patient portals. Still, these technologies have not been able to deliver on their promises due to their high costs, complex implementation processes, lack of interoperability among systems, etc.
The future of data science in healthcare will be driven by the rise of artificial intelligence (AI) and machine learning (ML) technology. These two technologies are already revolutionizing many industries, from finance to retail, so it’s not surprising that they are also finding their way into the healthcare industry.
Conclusion
Data science applications in healthcare are already benefiting society, and there is no doubt that they will be even more valuable in the upcoming era. It will advance the healthcare industry. Doctors will be well-served, and patients will benefit from a unique experience and optimal treatments.
Big data can help realize long-term visions for self-management, improved patient care, and treatment. Data science can provide real-time predictive analytics that can be used to gain insights into various disease processes and deliver patient-centered care. It will aid in advancing researchers’ scientific abilities, epidemiological studies, personalized medicine, and so on. On the other hand, predictive accuracy is highly dependent on efficient data integration obtained from various sources to be generalized.
Lastly, if you are looking for help with implementing any of the foresaid use cases using artificial intelligence, machine learning, or deep learning models, we can help. Whatever your needs are, we can help you with our data science and analytics services and become your reliable partner. Want to learn more? Talk to one of our experts.
Related Posts