Reading Time : 2 Mins
How Is Data Analytics Used in Business?
I write about fintech, data, and everything around it
Data analytics is an increasingly important aspect of business, and it’s also one of the most misunderstood. I hope that this blog can provide some helpful information about how data analytics is used in business.
Data analytics has been used throughout human history to interpret data to predict future trends. Whether it be observing animal behavior or the trends of ancient civilizations, humans have always looked for ways to distill large amounts of information into useful knowledge nuggets.
Today, the term “data analytics” is used in many ways, sometimes even interchangeably with more obvious terms like “business intelligence” or “market research.”
However, in an ideal world, all these terms are distinct yet complementary — each having its own unique benefits and characteristics. I believe that modern businesses would have greater success if they combined such AI & ML technologies in their business to achieve their goals effectively.
To help build a data analytics strategy, I’m writing this blog post to explain one particular use case: how data analytics is used in business (and why it matters).
In this blog post, you will get an overview on:
- What is data and business analytics?
- Why is data analytics important for business?
- What are the types of data analytics?
- How does data analytics work?
- Data analytics use cases in business
- Advantages of data analytics in business
Let’s get started.

What is data and business analytics?
Data and business analytics are often used interchangeably. However, data analytics is a subset of business analytics, which focuses on using data in analyzing current and past business performance to gain insights that help executives make better decisions. Business leaders may use data analytics, but it isn’t limited to just the C-suite. It can be used across all levels of the organization where decision-making is involved.
For example, a human resources manager may use data analytics to determine how internal processes change affects employee retention. Or a marketing manager may use data analysis to assess the impact of advertising campaigns on sales for individual product lines.
Many people think data analytics is only for big businesses. However, companies of all sizes can benefit from using data and analytics to improve their decisions.
It doesn’t matter if your company is big or small, established or just starting out — making informed decisions from big data will help improve operational efficiency, increase profitability, get customers, and keep them happy.
Why is data analytics important for business?
Data analytics allows a business to create reports and find patterns that can help it operate more efficiently. Analyzing data can also improve decision-making by enabling a company to predict trends in its industry or predict what its customers will want next. These predictions help companies stay on the cutting edge and remain competitive.
For example, a pizza shop may use analytics to understand the demographics of its customers or how much they spend at the restaurant. This can help them plan promotions and marketing campaigns better.
Data analytics can be helpful to understand the different types of customers who visit the store. For example, if the pizza shop discovers that most of its customers are families with children, it may want to offer more kid-friendly items. On the other hand, if students are regular customers, they may like to provide student discounts as marketing incentives.
The pizza shop might also use data analytics to assess employee performance based on sales data from each server. If one server has low sales throughout the day, his boss may want to check on him and see if he needs more training or is not performing up to standards.
In the era of big data, where business leaders have access to more information than ever before, analyzing this information is an important skill for any professional in a leadership role.
That’s why many employers make business analytics training a priority for their employees who are looking to advance within the company.
What are the types of data analytics?
The number of different types of data analytics is vast, but they all fall under one of four categories: descriptive, diagnostic, predictive, and prescriptive.

1. Descriptive analytics
Descriptive analytics focuses on what happened in the past. It doesn’t look forward, but it does provide a comprehensive picture of how events unfolded. The primary benefit of descriptive data analysis is that it helps people understand exactly what happened and why.
Some common examples include:
- Sales performance: In sales, managers might want to know when daily sales exceed a certain amount or how many units each employee has sold this month.
- Dashboard reporting: Many e-commerce companies uses dashboards for real-time updates on page views, unique visitors, and user sessions across its various properties worldwide.
- Fraud detection: Credit card companies constantly monitor transactions for suspicious activity that may indicate credit card fraud.
- Product demand forecasts: Retailers use historical sales data to predict how much inventory will be needed for future periods.
2. Diagnostic analytics
Diagnostic analytics seeks to answer why an issue occurred by looking at the factors that led to an event. This type of analysis can help companies understand not only what happened but also why it happened and how they can prevent it from happening again.
The most common examples of diagnostic analytics are:
Root cause analysis. Root cause analysis is an analytical process used to identify the underlying causes of negative events, such as defects in manufacturing.
Retrospective analysis: Retrospective analysis involves examining historical data to determine why certain events occurred. Like root cause analysis, it can help identify potential risks and prevent future occurrences.
Drill-down: Drill-down refers to navigating through layers of detail to find the underlying reasons for an event or situation. For example, a retailer might use drill-down analysis to discover that inventory levels are low because sales have increased in a particular region within the last six months.
Regression analysis: Regression involves using statistical methods to identify trends and relationships between variables to make predictions about future outcomes. Regression models include making assumptions about how variables behave (e.g., normal distribution) and how they relate to each other (e.g., linear relationship).
3. Predictive Analytics
Predictive analytics uses existing data to determine future outcomes or trends. Companies often use this method when developing new products or services because it gives them an idea of what customers will want in the future based on their past behavior.
Examples of Predictive analytics in business:
Direct Marketing: The ability to determine which potential customers are likely to respond to a marketing campaign.
Customer Pricing: The ability to determine the optimal price for a product or service based on underlying demand.
Retail Sales Forecasting – Accurately forecasting demand for products at the SKU-store level across multiple time periods for ordering inventory, managing out-of-stocks, determining markdown targets, and managing the supply chain.
4. Prescriptive analytics
The prescriptive analysis takes predictive analytics one step further by using past trends and data to recommend future actions. This type of data analysis is most helpful in optimizing resources and identifying new business opportunities, such as expansion.
Prescriptive analytics can be used to make decisions or provide recommendations that allow others to make better and faster decisions.
For example, a prescriptive model could recommend whether an organization should:
- Start a new product line, or end an existing one.
- Build a new factory, or close an existing one.
- Place a bid on a project, and if so, at what price.
- Hire more people in the sales department.
- Send a targeted ad to a particular customer.
Honestly, the primary question is no longer whether a company should use data analysis but which type of data analytics is best suited to a particular situation.
What is data analytics in business?
Data analytics in a business context refers to the process of collecting, processing, analyzing, and interpreting large volumes of data to extract meaningful insights, patterns, and trends that can inform and guide strategic decisions, improve operational efficiency, and drive overall business growth. It involves using various techniques, tools, and methodologies to transform raw data into actionable information that can be used to make informed choices and optimize various aspects of the business.
In essence, data analytics in business involves the following key steps:
1) Data Collection: Gathering relevant data from various sources, including customer interactions, sales transactions, operational processes, marketing campaigns, and external market data.
2) Data Processing: Cleaning, organizing, and preparing the data for analysis, ensuring its accuracy and consistency.
3) Data Analysis: Applying statistical, mathematical, and machine learning techniques to uncover patterns, correlations, and insights within the data.
4) Data Interpretation: Interpreting the results of the analysis to derive actionable insights and draw conclusions that can guide decision-making.
5) Decision Making: Using the insights gained from data analysis to make informed decisions that impact various areas of the business, such as marketing strategies, product development, resource allocation, and more.
6) Continuous Improvement: Monitoring and evaluating the outcomes of decisions based on data analytics, and refining strategies over time based on new data and insights.
Data analytics provides businesses with the ability to move beyond guesswork and intuition and make decisions based on concrete evidence. It helps businesses understand customer preferences, optimize operations, mitigate risks, identify growth opportunities, and stay competitive in a rapidly changing business landscape.
How does data analytics work?
Data analytics is the process of making sense of data.
The process starts with collecting data, finding patterns, and then using those patterns to make predictions. These predictions can be used to set goals or make decisions. In sales, for example, you may want to use data analytics to predict how many of a particular product you will sell next month. Knowing that number helps you set goals for your team and plan inventory.
To start, we collect data and put it into a database. We organize the data by finding patterns within it (this is called cleaning and organizing). Then, we look for connections between patterns and develop a model that explains these connections (this is called modeling).
Once we have a model that works well enough, we use it to make predictions (this is called testing). These predictions are communicated to decision-makers in the form of reports findings.
Data analytics process typically takes place in 4 stages:

1. Data collection
Companies collect enormous amounts of information about customers, vendors, sales, and other business functions. Data can be collected from customer surveys, sales receipts, social media comments, and company websites.
A data integration platform can merge and centralize business data with the help of data pipelines for ease of access, management, and business intelligence. During this stage, you collect relevant datasets from various sources (internal or external) required for your project from a data lake, warehouse, or data mart. This can mean anything from pulling together sales numbers from an SQL database or scraping user reviews from the web using Python scripts. Once you have identified the right datasets, you load them into your preferred tool (Excel/SQL/Python), where you can wrangle and clean the data as required.
2. Data organization and analysis
Once this information has been gathered, it must be organized to make it easier to interpret and analyze. Data can be organized manually or through software programs that store the information in databases.
3. Model development, testing, and deployment
The next stage involves building a model that best fits the data set using various methods. These models are then tested and deployed if they meet specific criteria (e.g., accuracy over 95%).
4. Communicating results to decision-makers
The final step involves presenting the findings to company executives or other leaders to use insights to make better choices regarding products, services, marketing strategies, and other business areas.
How Data Analytics help Businesses?
1) Informed Decision Making: Data analytics empowers businesses to make strategic decisions based on real-time insights and trends, minimizing reliance on intuition and guesswork.
2) Identifying Opportunities: By analyzing large datasets, businesses can uncover hidden opportunities, market gaps, and emerging trends, allowing them to seize new revenue streams.
3) Customer Insights: Data analytics provides a deep understanding of customer behavior, preferences, and buying patterns, enabling businesses to tailor products and services to specific needs.
4) Operational Efficiency: Businesses can optimize processes and workflows through data analysis, identifying bottlenecks, inefficiencies, and areas for improvement to streamline operations.
5) Risk Management: Data analytics helps in identifying potential risks and vulnerabilities by analyzing historical data, enabling proactive risk mitigation strategies.
6) Personalized Marketing: By analyzing customer data, businesses can create personalized marketing campaigns that resonate with individual preferences, leading to higher engagement and conversion rates.
7) Competitive Advantage: Leveraging data analytics can give businesses a competitive edge by staying ahead of market trends, responding swiftly to changes, and outperforming competitors.
8) Resource Allocation: Businesses can allocate resources more effectively by using data to understand which initiatives yield the best returns, ensuring optimal resource utilization.
9) Predictive Insights: Data analytics enables businesses to make predictions about future trends and outcomes, enhancing long-term planning and strategy formulation.
10) Performance Tracking: Businesses can monitor key performance indicators (KPIs) through data analytics, enabling them to measure success, identify areas for improvement, and adapt strategies as needed.
Data analytics use cases in business
The use of data analytics in business is becoming increasingly important. Here is a look at some common data analytics use cases adopted in various business functions.
1. Data analytics use cases in marketing & sales
Marketing and sales are the most popular areas where companies have implemented data analytics use cases. Both sectors benefit from the use of data in separate ways:
With the help of big data, marketing managers can now make more accurate decisions. They collect data from various sources, such as social media, email communication, and other platforms, to communicate with their customers to understand their needs and preferences better. Marketing specialists then analyze the collected data to improve the marketing strategy and drive more sales.
Data analytics can help salespeople sell more effectively. For example, with the help of data analytics, sales representatives can easily understand which products are most demanded by particular target groups and focus on selling them to increase their performance level.
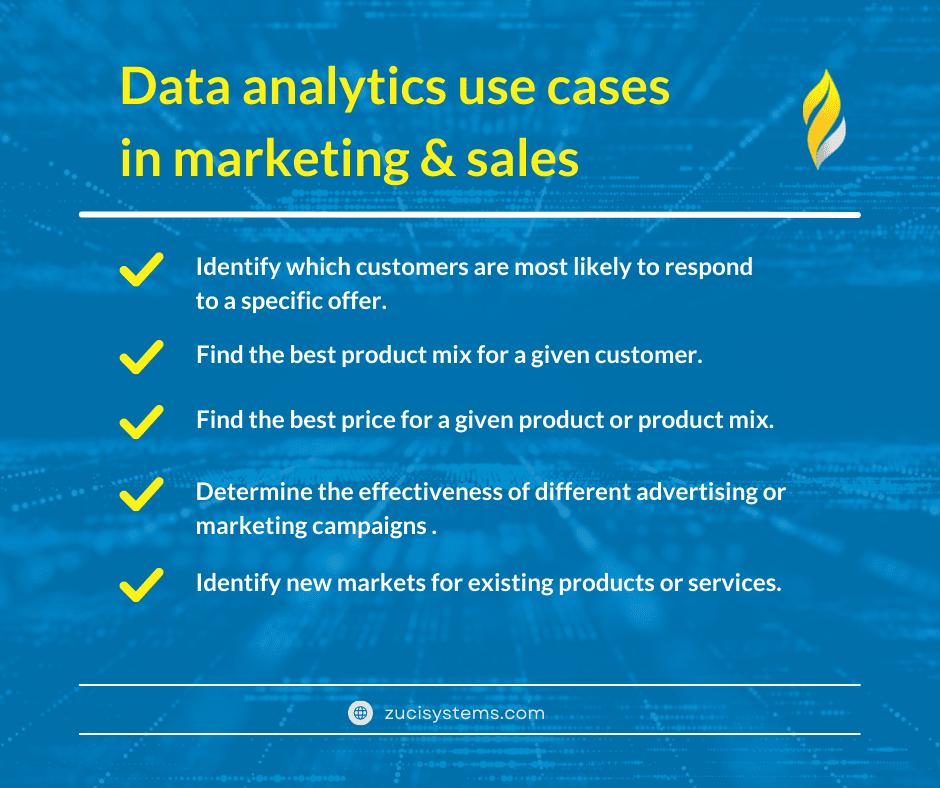
In marketing and sales, data analytics can help businesses to:
- Identify which customers are most likely to respond to a specific offer
- Find the best product mix for a given customer
- Find the best price for a given product or product mix
- Determine the effectiveness of different advertising or marketing campaigns
- Identify new markets for existing products or services
2. Data analytics use cases in operations and supply chain management
Any business with a supply chain or operations component can benefit from data analytics. For example, suppose you manufacture widgets, and those widgets require parts made by suppliers. In that case, you want to anticipate when your inventory is getting low so that you don’t run out of parts before they can be delivered. You might also use data analytics to optimize production schedules and staffing levels.
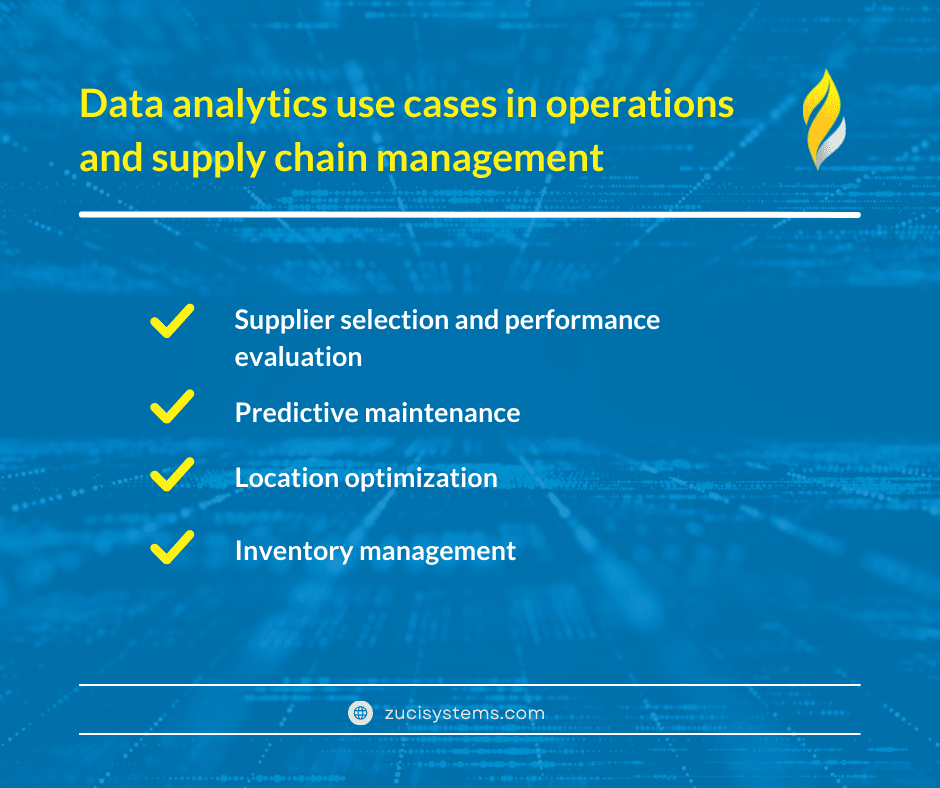
Here are some of the data analytics use cases in operations and supply chain management:
Supplier selection and performance evaluation: Using machine learning algorithms, supplier selection processes can be improved dramatically. In addition, companies can use data analytics to predict and manage supplier defaults.
Predictive maintenance: Predictive maintenance techniques enable companies to collect and analyze data from sensors to understand the state of equipment better and predict failures before they occur.
Location optimization: Companies can use location-based analytics solutions such as GIS (geographic information systems) to determine optimal locations for warehouses, factories, and service centers.
Inventory management: Data analytics can help companies better understand customer demand patterns to improve inventory control and reduce inventory costs.
3. Data analytics use cases in human resources
The use cases for data analytics in business are diverse, but one area ripe with opportunity is human resources.
A study by a popular research agency found that 84 percent of HR executives surveyed invest in analytics to improve talent management. Of the 716 companies surveyed, over half (56 percent) plan to increase analytics spending in the next two years.
Those investments are paying off: 63 percent of the companies surveyed said they already have a return on their investment in analytics.
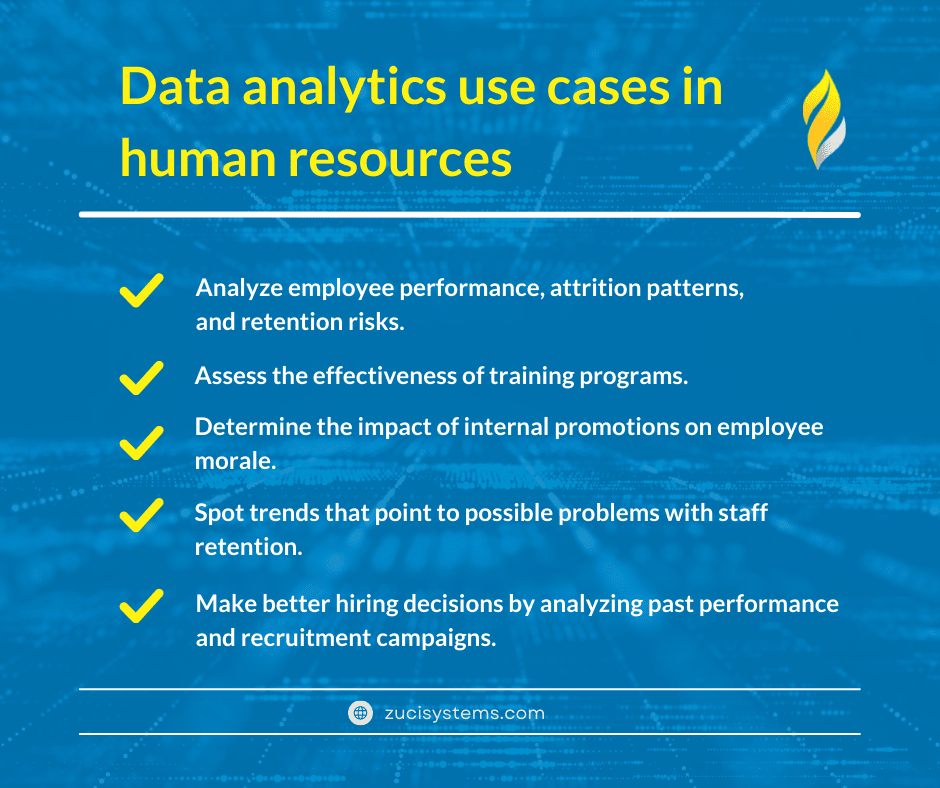
But how exactly do you use data analytics in human resources? Here are some of the use cases.
- Analyze employee performance, attrition patterns, and retention risks
- Assess the effectiveness of training programs
- Determine the impact of internal promotions on employee morale
- Assess training and development needs
- Spot trends that point to possible problems with staff retention
- Make better hiring decisions by analyzing past performance and recruitment campaigns to identify the best methods for attracting top talent
4. Data analytics use cases in customer service
The customer is king, and most companies want to understand their customers’ wants. Using data analytics and artificial intelligence, they can now get deeper insights into customer behavior.
Most businesses use data analytics in customer service in one form or another. It may be as basic as tracking metrics like number of calls or wait time on hold. But companies can also use data analytics to understand better customers’ needs, preferences, satisfaction levels, and more.

In the near future, you’ll likely see more companies take advantage of these types of data use cases:
- Identify common complaints and problems that customers have about products or services
- Resolve issues faster and more effectively through a better understanding of customer history and needs
- Predict what products or services a customer is likely to buy next
- Provide customers with personalized content and recommendations
- Automate processes such as payment processing and fraud detection
- Reduce the cost of delivering support, such as by providing self-service options
5. Data analytics use cases in finance
Finance is one of those departments that seems like it would have been made for big data — after all, financial data has been tracked by computers since there were computers. But actually, it’s quite likely that your finance department is still doing most of its work manually. And this means that it’s missing out on all sorts of opportunities for efficiency and innovation.
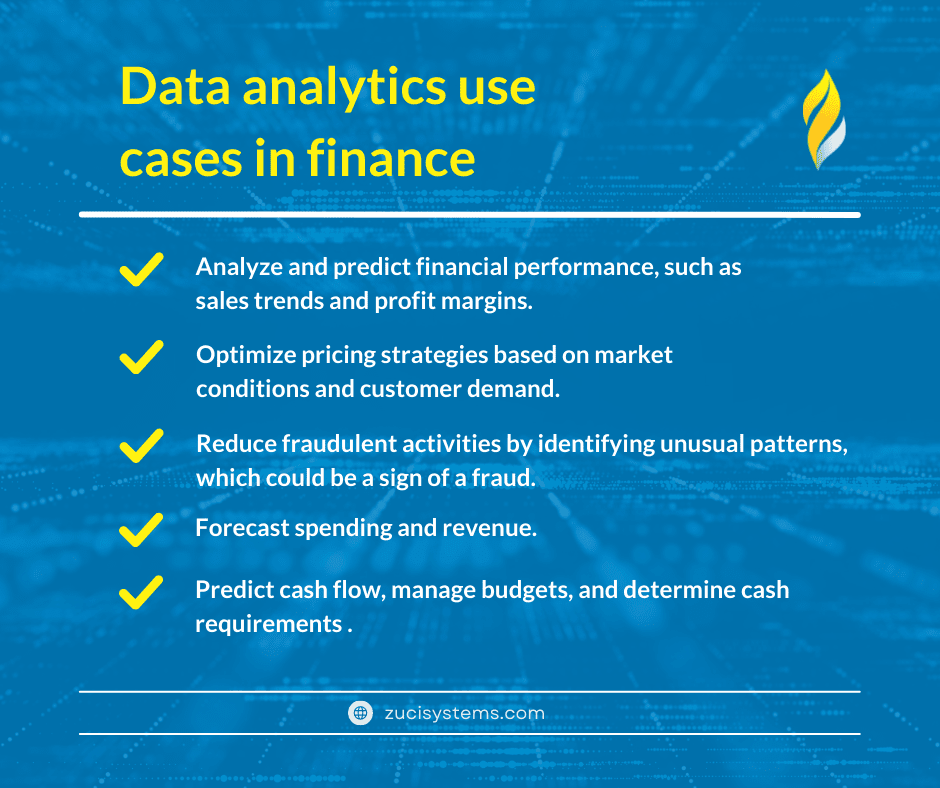
Here are some use cases of data analytics in finance departments:
- Analyze and predict financial performance, such as sales trends and profit margins
- Measure the efficiency of marketing campaigns and make informed decisions on where to put more money into and which one to cut back
- Optimize pricing strategies based on market conditions and customer demand
- Reduce fraudulent activities by identifying unusual patterns, which could be a sign of a fraud
- Forecast spending and revenue
- Predict cash flow, manage budgets, and determine cash requirements
Advantages of data analytics in business
According to Forbes, data analytics is a primary driver for most businesses today.
Businesses that use data analytics have a massive advantage over those who don’t. Data analytics can help you better understand your customers and their needs, leading to new business opportunities.

1. Reduce Costs
Using your company’s data information is a great way to save money and help your organization run more efficiently. One example: A freight company reached out to us for an integration center of excellence used its data to show that a particular lane was unprofitable for them, and they were able to eliminate that route. The company saved money by reducing the number of shipments on that specific route.
2. Improve Efficiency
Data analytics can help improve a company’s efficiency by helping them discover areas where they aren’t being efficient.
Data analytics allows businesses to collect large amounts of data, which can be analyzed and then used to identify weaknesses in the business model. Inefficiency is often a weakness that companies don’t notice right away because they are too focused on other things. However, inefficiency can cause a significant drain on profits and even lead to the business’s demise.
Efficiency is essential, but it isn’t always easy to determine where inefficiencies lie. That’s where data analytics comes in.
3. Make Better Decisions
One of the major advantages of data analytics in business is that it helps you make better decisions. Understanding what happened in the past, what’s happening now, and what might happen in the future can be a game-changer for your business.
Companies that use data analytics can predict customer behaviors and desires, making them more likely to provide services that customers want to consume. For example, a retailer may use data analytics to determine which products have been selling well and then order more of those products and similar items.
Another example is that Netflix uses data analytics to determine which TV shows and movies are most popular with its users. The company then decides which shows to produce based on this information. Some of their success in data analytics resulted in ‘Orange is the New Black’ and ‘House of Cards.’
4. Makes Your Business More Competitive
Data analytics give businesses a chance to get ahead of their competition by giving them better insight into their consumer base and how they can reach them. It can also help companies identify what they are doing wrong and how they can improve on it.
5. Increase Revenue
Data analytics can help businesses increase revenue by providing them with insights into how to make better decisions in terms of pricing and product offerings. For example, data analysis could indicate that most customers who buy one product also buy another particular product. A business could then decide to bundle these two items together and offer them at a lower price than if they were sold separately.
Takeaway
It is undeniable that data analytics has revolutionized the way businesses make decisions. The power of big data and analytics is in their capability to help businesses understand their customers, competitors, and other business elements better for business growth.
With proper data analytics, you can have a complete view of various aspects of your business with time, which would be impossible for an individual to monitor.
Whether you are running a small business/enterprise or large–scale organization, monitoring the data closely is the key to success in all cases. Schedule a 30-minute call with Zuci’s data engineers to build a single source of truth system for real-time business reporting, optimization, and analysis. Sign-up and get your customized roadmap for Free.
Related Posts