Reading Time : 1 Mins
How to structure data science team for your enterprise?
I write about fintech, data, and everything around it
In this article, you will gain a deeper understanding of and structure of a data science team, key models, and roles that you should consider while structuring a data-driven organization team.
Businesses are increasingly viewing data as a valuable asset that will benefit them now and in the future. Data and analytics were rated as significant contributors to the company’s growth. But with the rising volume of data, new challenges and complexities are also forming shapes. To overcome these challenges and complexities, you need a strong data science team that helps you tackle them all.
A data science team will assist you in efficiently turning that volume of data into valuable knowledge. You wouldn’t be too far off the mark if you compared them to a data magician. On a high level, a typical data science team combines traditional mathematical concepts like statistics and analytic thinking with newer technologies like Machine Learning (ML) and Natural Language Processing (NLP).
To help you gain a deeper understanding and structure of a data science team, we have included this definitive article that covers everything essential. The report also covers key models and roles you should consider while structuring a data science team.
Lets get started.
What are data-driven teams?
Data-driven teams are the ones that use data to make decisions. These teams can make the right decisions at the right time because they use data as information. The main purpose of data-driven teams is to increase efficiency, productivity, and quality. When you have a team that uses data, it means that you have a team that can make decisions with confidence.
A data-driven team uses data to decide how to run their business. They track their performance and use the information to guide their strategies and tactics. They may use surveys or other data collection forms to get information about people’s feelings about their products or services. They may also use statistics and other types of analytics to understand trends in customer behavior over time.
Data will be your friend if you want this kind of team. However, you should know how to use it correctly for it to be effective. A good example is when a marketing manager wants to know what type of content works best on social media platforms such as Facebook or Twitter. They need to use analytics tools like Google Analytics to find out what works best for their business model.
Key Roles & Models of the data science team
Team structure
The Data Science team structure isn’t one-size-fits-all. Every firm has varied data science needs and uses cases. Data science teams can be as listed below.
Key models | How it works |
Centralized | Centralized data scientists report to the department head in small teams. Centralized teams have more resource allocation options, and analysts gain problem-solving experience. A structured model may document and scale best practices for consistent results and simple teamwork. |
Decentralized | Data scientists in a decentralized organization report directly to the advertising or marketing content departments. In larger or medium-sized firms, the decentralized model takes shape since individual departments can hire and manage their own data science team in addition to an adequate need in data science use cases. |
Hybrid | Hybrid teams blend decentralized and centralized organizations. The manager thinks of the data science team as a collective entity, yet each member works on business processes or divisions. |
Democratic | This structure decentralizes the team’s data and gives everyone in the organization portal access. This increases transparency, communication, and teamwork with executives and stakeholders. |
Consulting | This structure divides the team into consultants who help with business demands or departments. This reduces centralized system costs and improves task requests. |
Functional | The functional structure allocates the data science team’s resources and capabilities to one functional department. This structure is appropriate for startups that will need simple data analysis. |
Federated | This structure sends data science professionals to specific assignments or other areas of the company while an analytics team works from the CoE. This paradigm blends decentralization with cooperation for a more efficient team. |
Center of Excellence (CoE) | This strategy designates a single center of excellence to manage data analytics inside the corporation. This improves team quality and expands data science activities. |
Data Science Team Roles
In the world of data science, many different roles exist. From the person who collects and cleans the data to the person who interprets it, there are many opportunities for you to get involved in this exciting field. Let us examine some of these roles suggested by Stitch Fix’s Michael Hochster. Michael distinguishes two types of data scientists: Type A and Type B.
Type A – Analysis
The data science team is responsible for analyzing the data we use to make decisions and predictions. They use various techniques and technologies to figure out what’s happening in our business, how it’s changing over time, and what that means for us now and in the future.
Type B – Building
The functions of the Building Data science team are as follows:
- Data scientists are responsible for interpreting data, identifying trends and patterns, and making recommendations to management. They also help establish processes and procedures for collecting, storing, analyzing, and disseminating data.
- Data analysts work with business managers to create reports based on research and analysis that help make decisions.
- Business intelligence (BI) professionals use software programs such as SPSS to analyze data sets that can be used to make business decisions. They also make dashboards that show key performance indicators (KPIs) so that executives can track how well their company is doing at meeting its goals and objectives.
- Data engineers build systems that allow companies to store large amounts of information in databases while ensuring that it’s safe from loss or corruption due to hacking attempts by third parties who may want access to sensitive information about customers or suppliers whose names are stored on servers owned by another business entity located within city limits (such as Walmart).
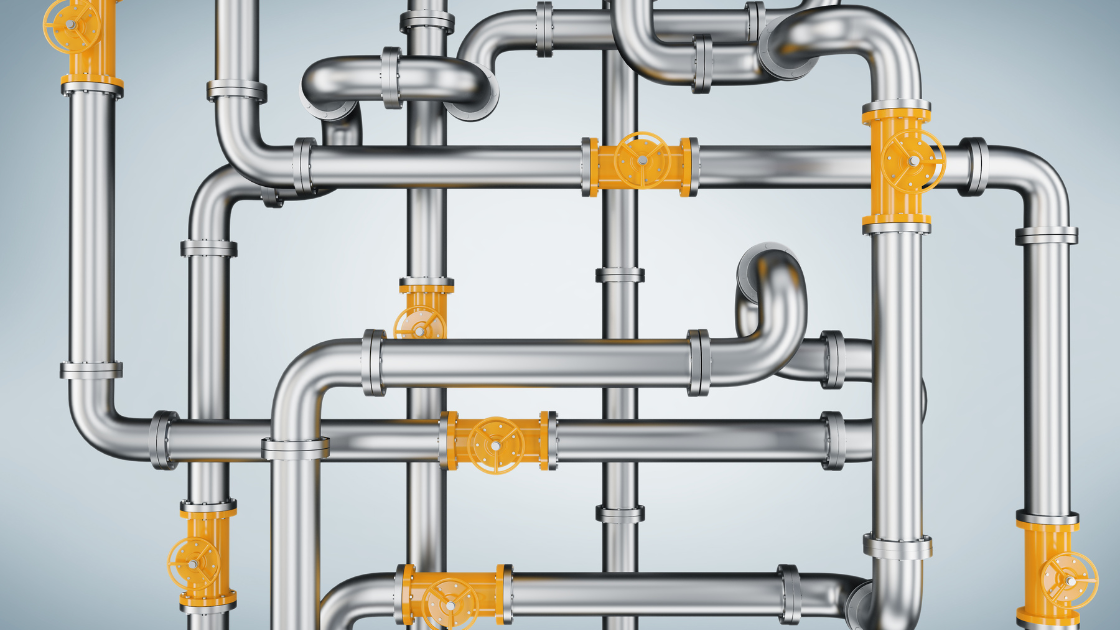
Data Pipeline Components, Types, and Use Cases
Even the most seasoned data scientists can rapidly become overwhelmed by the significant data volume, velocity, and diversity. Check out this article on data pipelines that covers different data pipeline components, types, use cases, and everything around it.
Key players on a data science team
Active Roles In A Data Science Team | What Way They Perform |
Chief Data Officer/Chief Analytics Officer | The CAO and CDO oversee all data-related efforts. CAOs focus on analytics whereas CDOs focus on data. |
Data Engineer | Data engineers will add new data sources to systems easily and fast. They’ll also make sure team members’ code meets management or project standards. |
Data Analyst | Analysts make decisions after studying analytics or BI data.
Reporting, dashboards, and data mining are done. Dashboards give clients real-time web or mobile app data access, while reports employ current datasets. “Data mining” employs algorithms to find patterns in massive amounts of data, generally financial activities. |
Data Scientist | Team-based data scientists interpret data. They build predictive models and analyze data. Data analysis helps companies make better decisions by forecasting future patterns or outcomes.
A data scientist knows business, statistics, and math. |
Business Analyst | A business analyst examines a company’s needs and requirements to develop solutions. They need stakeholder interviews, user research, or test runs. Using this data, document the solution and how it meets the criteria. |
ML Engineer | A machine learning engineer develops and applies methods that let computers learn from data. They create computer algorithms that can make predictions without being told how. They work with enormous, diverse datasets. |
What kinds of industries need data scientists?
1. Financial data science
Banking and finance value data science. Credit risk modeling, fraud detection, and customer segmentation are high priorities in Banking and Finance (customer profiling based on behavior and characteristics for personalization of offers and services. Finally, real-time predictive analytics uses data science.
2. Hospitality Data Science
Data analytics helps hotels with pricing strategies, consumer analysis, brand marketing, and more.
Airbnb uses data science to analyse customer feedback. Airbnb aggregates individual experiences to generate community patterns. Data science trends impact company decisions and help them expand.
3. Data science and the tourism industry
These businesses may boost personalization and user interactions by utilizing recommendation engines informed by data science. The sentiment analysis of social media posts is another area where data science is used to provide really helpful insights linked to travel.
4. Healthcare data science
AI is transforming the healthcare industry. Medical imaging and data science help doctors make better diagnoses and treat patients. Advanced health informatics tools are developed to improve patient care. These techniques help define tailored patient prescriptions, decreasing clinic and hospital operational costs. Natural Language Processing (NLP) is often utilized in healthcare to analyze textual research data.
5. Logistics data science
Big data and predictive analytics drive Supply chain innovation. They increase company visibility, cut expenses and overheads, estimate demand, do predictive maintenance, price products, prevent supply chain interruptions, optimize routes, manage fleets, and more.
How to Integrate a Data Science Team Into Your Company?
Integrating a data science team into your company can be much smoother than you might think.
Data science is a field that’s still relatively new, and as such, there are no real rules on how to integrate it into your company. However, there are certain ways that you can make sure you’re doing it right—and here are a few tips to help you out:
1) Ensure that everyone understands the importance of data science. If everyone on your team doesn’t understand the value that data science can provide for your company, then there will be no way for them to integrate it into their work truly. This means communicating with your team about what data science does and why it matters for your business.
2) Make a slow transition. You don’t want to rush into integrating data science because it’s new! Instead, focus on one aspect of the process at a time, starting small and working up from there. This will help ensure that everyone feels comfortable with the changes being made before they’re introduced too quickly or pushed too far too fast.
Final Thoughts
Businesses around the world have now become more aware of how data can be a significant competitive advantage. They now use data in many ways. Some are only beginning. Others have already established their whole data expert teams. And as the need for managing and utilizing data increases, the issue of how to structure the data team will undoubtedly arise with it. We recommend you look for a reasonable ROI of ML investment because it exists.
We hope you like this article and learn how data labeling is an intrinsic part of data science! Book a discovery call for our data science and analytics services today and get ahead of the competition. Make it simple & make it fast.
Related Posts